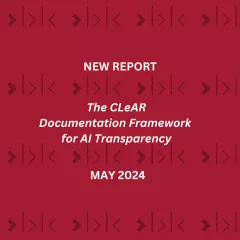
New Report: Framework for AI Transparency
Recommendations for Practitioners & Context for Policymakers
Summary
With growing attention to AI regulation, rights-based principles, data equity, and risk mitigation, this is a pivotal moment to think about the social impact of AI, including its risks and harms, and the implementation of accountability and transparency. Transparency can be realized, in part, by providing information about how the data used to develop and evaluate the AI system was collected and processed, how AI models were built, trained, and fine-tuned, and how models and AI systems were evaluated and deployed. Towards this end, documentation has emerged as an essential component of AI transparency and a foundation for responsible AI development. The full report, co-authored by experts across academia, industry, and civil society, is available at the Shorenstein Center website.
This report introduces the CLeAR Documentation Framework, designed to help practitioners and policymakers understand what principles should guide the process and content of AI documentation. The report introduces four principles for documentation and offers definitions, recommends approaches, explains tradeoffs, highlights open questions, and helps guide the implementation of documentation.
The CLeAR Principles state that documentation should be:
- Comparable: Able to be compared; having similar components to documentation of other datasets, models, or systems to permit or suggest comparison; enabling comparison by following a discrete, well-defined format in process, content, and presentation.
- Legible: Able to be read and understood; clear and accessible for the intended audience.
- Actionable: Able to be acted on; having practical value, useful for the intended audience.
- Robust: Able to be sustained over time; up to date.
Authors
Kasia Chmielinski* (Data Nutrition Project & Harvard University)
Sarah Newman* (Data Nutrition Project & Harvard University)
Chris N. Kranzinger* (Data Nutrition Project)
Michael Hind (IBM Research)
Jennifer Wortman Vaughan (Microsoft Research)
Margaret Mitchell (Hugging Face)
Julia Stoyanovich (New York University)
Angelina McMillan-Major (independent researcher)
Emily McReynolds (independent researcher)
Kathleen Esfahany (Data Nutrition Project & Harvard University)
Mary L. Gray (Microsoft Research, Indiana University & Harvard University)
Maui Hudson (TKRI, University of Waikato)
Audrey Chang (Data Nutrition Project & Harvard University)
*Joint first authors