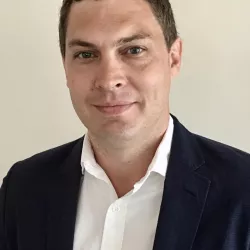
Ryan Copus's primary research interest is in machine learning as a tool to study, evaluate, and improve the administration of justice, with a focus on civil procedure, federal courts, and expert evidence. He completed his Ph.D. at Berkeley Law School’s Jurisprudence and Social Policy Program in 2017, with specializations in economics and biostatistics. His dissertation, “Machine Learning and the Reliability of Adjudication,” developed predictive models of decision-making in the United States Court of Appeals for the Ninth Circuit Court and the California Board of Parole Hearings, using them to investigate the promises and limitations of algorithm-guided justice. Ryan graduated with a B.A. in philosophy and sociology from the University of Texas before receiving his J.D. from Harvard Law School in 2007. Following law school, he clerked for the Honorable David C. Godbey of the Northern District of Texas and was a litigation associate for Goodwin Procter LLP.