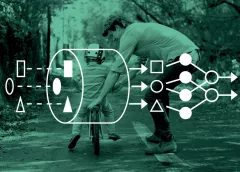
In this piece for Wired, Joi Ito explains why it's important to value the role of intuition in science.
For decades, artificial intelligence with common sense has been one of the most difficult research challenges in the field—artificial intelligence that “understands” the function of things in the real world and the relationship between them and is thus able to infer intent, causality, and meaning. AI has made astonishing advances over the years, but the bulk of AI currently deployed is based on statistical machine learning that takes tons of training data, such as images on Google, to build a statistical model. The data are tagged by humans with labels such as “cat” or “dog”, and a machine’s neural network is exposed to all of the images until it is able to guess what the image is as accurately as a human being
One of the things that such statistical models lack is any understanding of what the objects are—for example that dogs are animals or that they sometimes chase cars. For this reason, these systems require huge amounts of data to build accurate models, because they are doing something more akin to pattern recognition than understanding what’s going on in an image. It’s a brute force approach to “learning” that has become feasible with the faster computers and vast datasets that are now available.
You might also like
- storyIn AI We Trust?